이 글은 필자가 David Silver의 Reinforcement Learning 강좌를 듣고 정리한 글입니다.
🧵 Sequential decision making
- Goal: select actions to maximize total future reward
- To maximize total future reward, there might be tasks that long-term reward matters, so it may be better to sacrifice immediate reward to gain more long-term reward. Reward may be delayed.
- Solution of Sequential decision making
- Reinforcement Learning
- Planning
🧵 Characteristics of Reinforcement learning
What makes reinforcement learning different from other machine learning paradigms?
- There is no supervisor, only a reward signal
- Solution of Sequential decision making
- Feedback is delayed, not instantaneous
- Time really matters (sequential, non i.i.d data): Agent's actions affect the subsequent data it receives
🧵 Agent and Environment
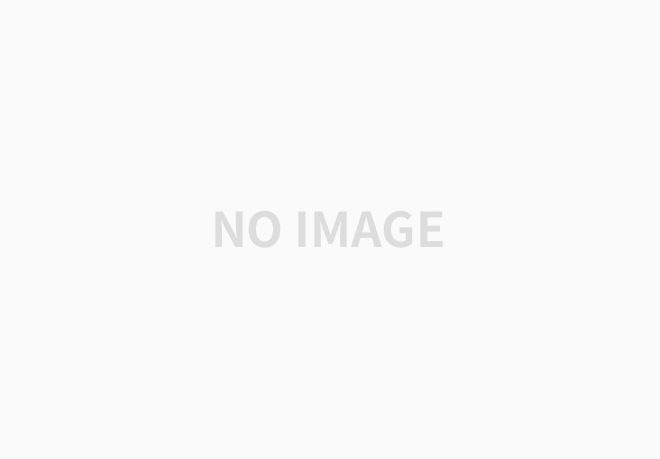
- Basic concept
- Exploration and Exploitation: trial-and-error learning
- Prediction (Given a policy, evaluate the future) and Control (Find the best policy)
- At each step tt, the environment,
- Emits observation OtOt
- Emits scalar reward RtRt
- On step tt, agent gets scalar feedback signal RtRt, agent wants to maximize cumulative reward.
- With the reward hypothesis: All goals can be described by the maximisation of expected cumulative reward.
- Receives action AtAt from agent
- Agent should include one or more of these components:
- Policy: agent's behavior function, a map from state to action. Can be deterministic a=π(s)a=π(s) or stochastic π(a|s)=P[At=a|St=s]π(a|s)=P[At=a|St=s]
- Value function: how good is each state is, a prediction of future reward. (Therefore it related to select better action.) vπ(s)=Eπ[Rt+1+γRt+2+γ2Rt+3+⋯|St=s]vπ(s)=Eπ[Rt+1+γRt+2+γ2Rt+3+⋯|St=s]
- Model: What the environment will do next based on the transition model and reward model:
- Transition model Pass′=P[St+1=s′|St=s,At=a]Pass′=P[St+1=s′|St=s,At=a]
- State Transition Matrix PP: For a Markov state ss and successor state s′s′, the state transition probability is defined by Pss′=P[St+1=s′|St=s]Pss′=P[St+1=s′|St=s]. State transition matrix PP defines transition probabilities from all states ss to all successor states s′s′
- Reward model Ras=E[Rt+1|St=s,At=a]Ras=E[Rt+1|St=s,At=a]
- Transition model Pass′=P[St+1=s′|St=s,At=a]Pass′=P[St+1=s′|St=s,At=a]
- History can be expressed on step tt: Ht=O1,R1,A1,⋯,At−1,Ot,RtHt=O1,R1,A1,⋯,At−1,Ot,Rt
- We additionally define state: the information used to determine what happens next based on the history St=f(Ht)St=f(Ht)
- Division of State
- Environment state SetSet is the environment's private representation. Usually not visible to the agent.
- Agent state SatSat is the agent's internal representation. information used by reinforcement learning algorithm
- Information state (a.k.a Markov state) contains all the useful information from the history. A state StSt is Markov if and only if P[St+1|St]=P[St+1|S1,⋯,St]P[St+1|St]=P[St+1|S1,⋯,St], "The future is independent of the past given the present." The state captures all relevant information from the history. i.e. The state is a sufficient statistic of the future.
- Observability
- Full observability: If agent directly observes environment state Ot=Sat=SetOt=Sat=Set, this is a Markov decision process (MDP)
- Partial observability: agent indirectly observes environment Sat≠Set, this is partially observable Markov decision process (POMDP). On this setting, agent construct its own state representation Sat based on history Ht, Beliefs of environment state (P[Set=s1],⋯,P[Set=sn]) or Recurrent neural network σ(Sat−1Ws+OtWo)
- Division of State
🧵 Categorizing RL agents
- ~ Based
- Value Based: Value function
- Policy Based: Policy
- Actor critic: Policy + Value function
- Model related
- Model Free: Policy and/or Value function
- Model Based: Model + Policy and/or Value function
'Robotics & Perception > Reinforcement Learning' 카테고리의 다른 글
[David Silver] 5. Model-Free Control: On-policy (GLIE, SARSA), Off-policy (Importance Sampling, Q-Learning) (0) | 2022.04.09 |
---|---|
[David Silver] 4. Model-Free Prediction: Monte-Carlo, Temporal-Difference (0) | 2022.04.09 |
[David Silver] 3. Planning by Dynamic Programming (0) | 2022.04.02 |
[David Silver] 2. Markov Decision Processes (0) | 2022.04.02 |
[Basic] 헷갈리는 용어 정리 (0) | 2022.01.04 |